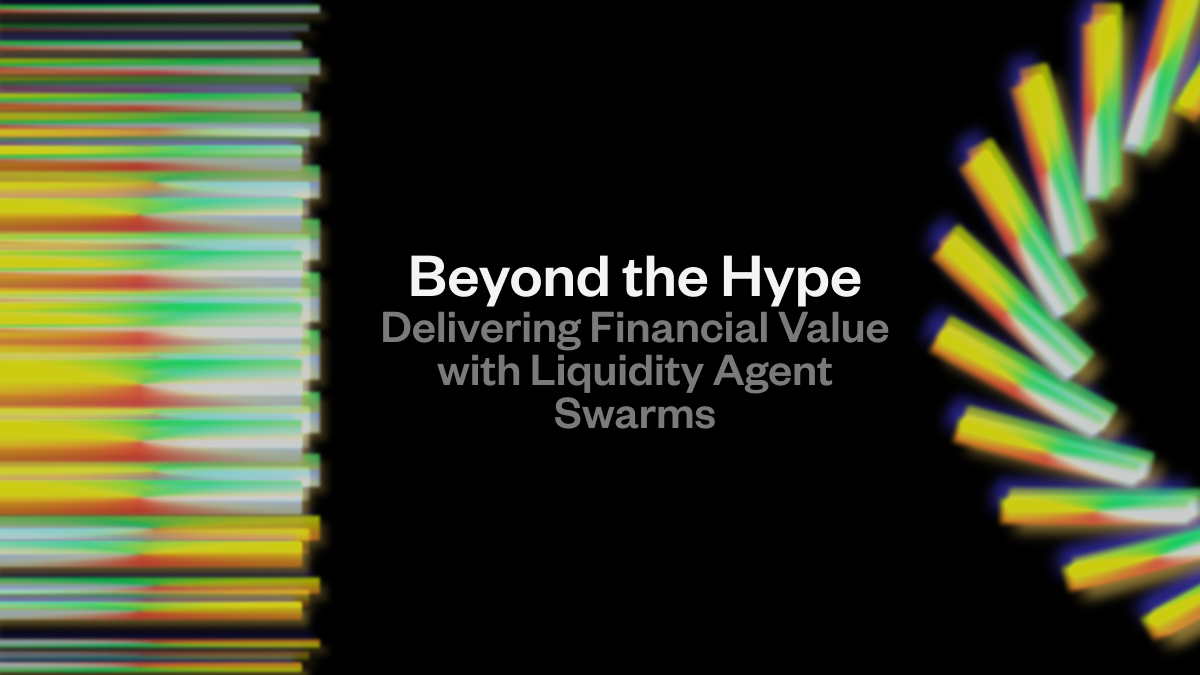
The intersection of artificial intelligence (AI) and decentralized finance (DeFi) is still in its early stages, yet the potential impact is undeniable. Theoriq is at the forefront of exploring how AI agents coordinating as swarms can utilize large language models (LLMs), reinforcement learning (RL), and other advanced methodologies that can be integrated into DeFi to optimize liquidity provisioning (LP) and strategic decision-making.
Integrating AI into DeFi introduces dynamic, agent-based models that can adapt in real time to evolving market conditions. This is core to advancing the agentic economy, where we believe it is essential to develop agent swarms that participate as first-class, native actors within DeFi ecosystems.
Within the agentic economy, liquidity stands as one of the key “layers” of the stack. A fundamental challenge in this layer is liquidity provisioning, traditionally a highly temporal process that requires continuous monitoring and decision-making based on market data. The introduction of AI-powered agents at this level can greatly enhance LP by developing strategies that adapt to changing market conditions in real time.
Reinforcement learning (RL), a well-established AI technique, provides a robust framework for tackling this problem.
In traditional AI research, reinforcement learning is frequently used to develop agents that can navigate complex environments by learning optimal policies through trial and error. Similarly, LP in DeFi can be framed as a Markov decision process (MDP), where an agent observes market conditions and determines optimal actions to maximize returns. In this setup, agents learn the optimal liquidity placement based on key market data like trading volume, volatility, and price movement.
Reinforcement learning, where agents learn optimal strategies through repeated interactions with an environment, has been widely applied in various domains, including robotics, drug discovery and powering the emerging class of reasoning AI models. The same principles can be applied to DeFi, where an agent must make decisions that influence subsequent market dynamics. The goal is to develop a policy—a predefined set of rules that dictate how an agent should act under specific market conditions.
LP is an ongoing optimization problem with constantly shifting variables, and Theoriq treats this as a RL challenge. Our swarms of agents learn and adapt based on real-time market dynamics, offering a competitive advantage over static AMM models that can’t adjust to changing liquidity needs.
This research feeds into the Theoriq Protocol which abstracts liquidity management into a structured learning problem. Instead of relying on manually coded policies, Theoriq is exploring ways that agents can autonomously generate and refine liquidity strategies autonomously. This involves:
While traditional liquidity provisioning relies on static strategies and heuristic-based decision-making, AI-driven approaches offer a dynamic and data-responsive alternative with several key advantages. Agents are capable of adaptive learning, continuously analyzing new market data to refine and adjust their strategies in real time. This responsiveness allows them to remain effective in rapidly changing market conditions.
AI systems can also enhance forecasting by integrating a wide range of signals, from price movements to broader macroeconomic trends; this enables more accurate anticipation of market shifts. By simulating various LP strategies, AI systems can identify the most efficient execution paths, helping reduce costs and maximize returns. The autonomous nature of agents streamlines operations by minimizing the need for human intervention, improving overall system efficiency.
Large language models (LLMs) also play a key role in the Theoriq’s protocol. These models are capable of interpreting complex academic research on LP, extracting key policy insights that inform strategic development. By translating these insights into executable code, LLMs can help generate baseline policies that serve as the foundation for complex strategies.
LLMs also enhance the decision-making capabilities of agents through advanced natural language processing. This allows for more nuanced refinement of policy functions, leading to smarter, more adaptive strategies in DeFi.
Theoriq’s strategy for integrating efficient agent swarms into DeFi follows a structured roadmap aimed at delivering practical, incremental progress. It begins with the development of baseline liquidity agents built using policies that are deterministic, which will be key to bootstrapping the system. With these foundations established, the next phase focuses on enhancing forecasting capabilities by training AI models to incorporate predictive analytics into their decision-making processes.
As the system evolves, reinforcement learning techniques are implemented to optimize performance. This means agents can continuously refine their strategies through backtesting and real-time simulations. The final stage involves deploying execution agents within live DeFi protocols, where their effectiveness is closely monitored, evaluated, and improved through ongoing iterations.
Agents that integrate these innovations play a crucial role in transforming the capabilities of AI models into real-world value. By creating tangible financial value for users, projects, and specific use cases, they help make DeFi more efficient, adaptive, and dynamic, bridging the gap between advanced technology and practical utility.
While still in its early stages, the fusion of agent swarms with DeFi presents immense potential. Much of this remains in the research and experimental phase, however, the hypotheses and initial implementations within Theoriq’s protocol point toward a high likelihood of success.
If agent swarms prove effective in onchain liquidity provisioning, they could fundamentally reshape the entire crypto stack—enhancing active trading, market making, and yield optimization. This progress lays the groundwork for a new economic model and agentic economy—driven by intelligent agents and dynamic liquidity strategies. Theoriq is paving the way for this future, ensuring that agent swarms enhance the efficiency, transparency, and profitability of decentralized financial systems.
The AI Revolution will not be Centralized! Join Theoriq on a mission to govern AI through responsible, inclusive, and reflectiv…
Theoriq is committed to building a responsible, inclusive, and consensus-driven AI landscape in Web3. At the forefront of integrating AI with blockchain technology, Theoriq empowers the community to leverage cutting-edge AI Agent collectives to improve decision-making, automation, and user experiences across Web3.
Theoriq is a decentralized protocol for governing multi-agent systems built by integrating AI with blockchain technology. The platform supports a flexible and modular base layer that powers an ecosystem of dynamic AI Agent collectives that are interoperable, composable and decentralized.
By harnessing the decentralized nature of web3, Theoriq is unlocking the potential of collective AI by empowering communities, developers, researchers, and AI enthusiasts to actively shape the future of decentralized AI.
Theoriq has raised over $10.4M and is backed by Hack VC, Foresight Ventures, Inception Capital, HTX Ventures and more, and have joined start-up programs with Google Cloud and NVIDIA.