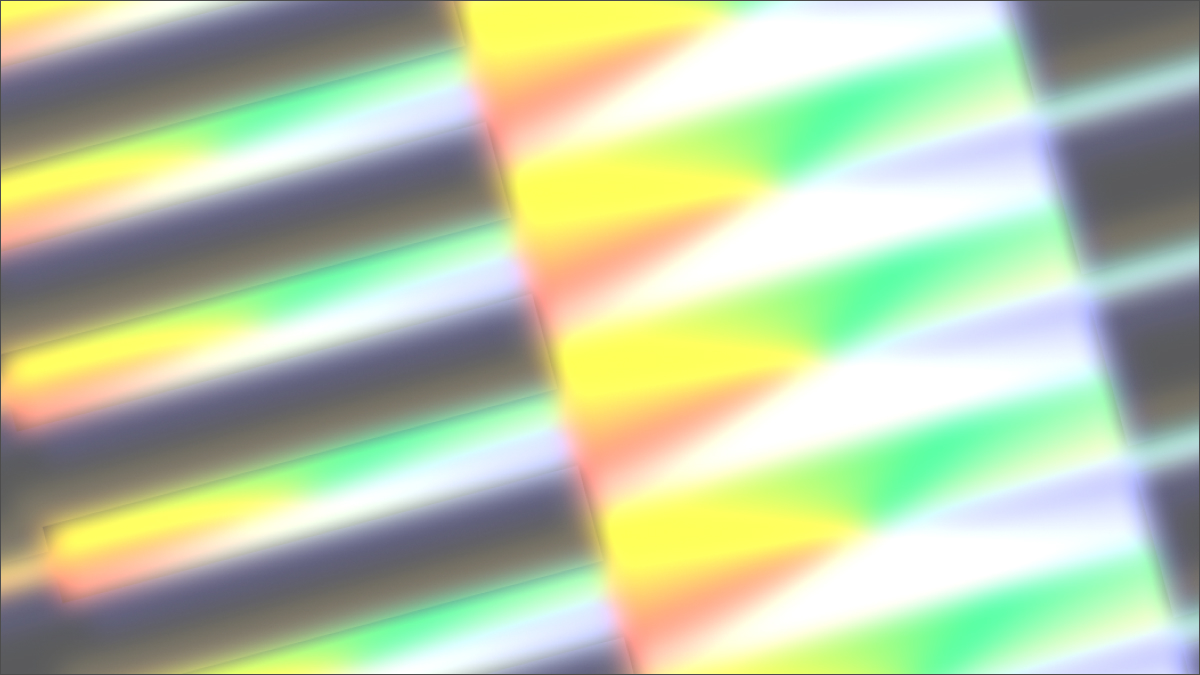
The second week of the Theoriq Educational AMA series was another success, with many of our community members enjoying the engaging topics with our Head of AI Education Shingai Manjengwa and her team, Senior AI Solutions Engineer, Pourya Vakilipourtakalou and Alexander Marx.
This week's series started with a casual chat about the teams experience at the Futurist Conference in Toronto last week, and then dove deeper into the Theoriq protocols three core pillars, the mechanisms behind agent selection, the role of evaluators and optimizers, and the exciting potential of building specialized AI agents, particularly in the domains of education and financial planning. Below is a summary of what was discussed.
Enjoy!
The Theoriq protocol, utilizing its AI Agent Base Layer has been developed to act as the foundational layer for enabling the efficient operation of multi-agent systems, also known as Agent Collectives – networks of AI Agents that collaborate to perform complex tasks. These systems are particularly valuable for specialized tasks that require the combined expertise of multiple agents, each with a unique set of skills.
An interesting topic to think about in the early stages of AI Agent development is the importance of responsible AI governance. As multi-agent systems become more sophisticated and autonomous, it's important to ensure that these agents operate within ethical boundaries and are accountable for their actions. This is where the principles of AI governance come into play, providing a framework for managing AI agents in a way that is transparent, fair, and aligned with societal values.
The Theoriq protocol is designed to create the fairer development of AI systems, steering the technology toward a more positive future. The AI Agent Collectives are built on blockchain which ensure more transparency, security and accountability. The protocol is flexible, modular and has a dynamic marketplace that is designed to evolve with AI progress and community needs, built upon three core pillars.
Interoperability Agents: this ensures that different agents can communicate and collaborate effectively across a diverse range of frameworks and models. This is done by giving developers the tools they need to easily build and customize agents, allowing them to work together without needing special permissions or restrictions.
Composable Collectives: this refers to the ability to combine different AI agents, each specialized in a particular task, to perform more complex operations. This is made possible by creating mechanisms to find, assess, and combine different agents into stronger collectives, with a system that tracks how well they do, helping them improve over time.
Decentralized Innovation: this is an integral part in ensuring accountability and transparency in AI Agents. This happens by creating a community-driven marketplace where developers, users, and agents interact, and are incentivized by token economics to continuously improve and expand the capabilities of the network.
How would these core pillars interact in a real world use case?
Imagine having an AI Agent Collective designed for financial market analysis. Multiple AI agents would be required to handle specific tasks throughout the process. Interoperability would be crucial, allowing these agents to share information and determine which ones are best suited for analyzing specific tasks like news feeds, social media trends, or data analytics. A modular and composable Collective would enable developers to create AI systems by seamlessly "snapping together" various agents, similar to building with Lego blocks. And running this on a decentralized structure allows for a community-driven and incentivized AI system that can continuously improve and evolve to meet users' needs.
In order for AI systems to work together effectively, Theoriq have introduced the following key components in their protocol architecture to enable the creation, interaction, and continuous evolution of AI Agents and Collectives.
Evaluators: these are specialized Agents dedicated to producing quality signals that contribute to Agents reputation and further influence their visibility in the protocol. These signals are key to helping users discover the best performing Agents for their specific needs.
Once evaluators have fulfilled their assessment, they submit a Proof of Contribution, this proof is issued to agents that have directly contributed to the successful completion of a task. It acts as a record of the agent’s performance, providing a trackable history of its contributions across various tasks. This proof is essential for maintaining accountability within the AI system, as it allows for the monitoring and evaluation of individual agent performance over time.
Optimizers: specialized Agents designed to enhance the performance of Collectives by identifying optimal Agent formations.
Once Optimizers have chosen the best performing collectives they submit a Proof of Collaboration, this proof is awarded to agents that have successfully worked with other agents to achieve the task’s objectives. Collaboration is a critical component of AI systems, especially for complex tasks that require the integration of multiple specialized agents. The proof of collaboration ensures that agents are not only performing well individually but are also capable of working effectively as part of a team.
These components are responsible for assessing the quality of agent outputs and selecting the best candidates for specific tasks. This process is key for creating an optimal collective of agents that can collaborate to complete sophisticated tasks. Currently there is a routing system powered by an untuned large language model (LLM), which helps in selecting the best agents based on past performance.
Human evaluators are also key to this process and complement AI evaluators by providing contextual understanding, ensuring a robust and effective agent selection process that combines the strengths of both AI and human insights.
One of the most exciting uses of AI is the potential to create specialized agents that cater to specific domains. In last week's AMA, the team highlighted the growing interest in building agents tailored to education and financial planning.
What could these Agents look like?
Educational Agents: these agents are designed to assist with learning and knowledge dissemination. They can be used to create personalized learning experiences, provide real-time feedback, and even offer tutoring in specialized subjects. The development of educational agents represents a significant step forward in making high-quality education accessible to a broader audience.
Financial Planning Agents: these agents are focused on helping users manage their finances more effectively. They can analyze spending patterns, provide investment advice, and create customized financial plans. Financial planning agents have the potential to democratize access to financial expertise, empowering individuals to make more informed decisions about their money.
The Theoriq protocol provides a forward-thinking approach that ensures AI systems are built using transparency, accountability, and ethical governance. Through interoperability, composability, and decentralization, Theoriq strengthens AI systems' functionality and adaptability while aligning them with societal values.
Evaluators and optimizers play key roles in maintaining the integrity of these systems, using their Proof of Contribution and Proof of Collaboration mechanisms. Theoriq's decentralized, community-driven platform supports dynamic AI systems and specialized use cases. As AI advances, Theoriq's innovative approach will be essential in steering the tech towards a positive future.
Make sure to tune into our next Weekly AMA Educational series on Discord and X.
If you want to dive deeper into all of the above, head over to our litepaper and read how we are building the future of AI Agent Collectives.
The AI Revolution will not be Centralized! Join Theoriq on a mission to govern AI through responsible, inclusive, and reflectiv…
Theoriq is committed to building a responsible, inclusive, and consensus-driven AI landscape in Web3. At the forefront of integrating AI with blockchain technology, Theoriq empowers the community to leverage cutting-edge AI Agent collectives to improve decision-making, automation, and user experiences across Web3.
Theoriq is a decentralized protocol for governing multi-agent systems built by integrating AI with blockchain technology. The platform supports a flexible and modular base layer that powers an ecosystem of dynamic AI Agent collectives that are interoperable, composable and decentralized.
By harnessing the decentralized nature of web3, Theoriq is unlocking the potential of collective AI by empowering communities, developers, researchers, and AI enthusiasts to actively shape the future of decentralized AI.
Theoriq has raised over $10.4M and is backed by Hack VC, Foresight Ventures, Inception Capital, HTX Ventures and more, and have joined start-up programs with Google Cloud and NVIDIA.